Insight Partners had a banner year in 2021, with more than $50 billion in capital commitments across over 200 investments. While we invest in founders across a wide spectrum of businesses, this series focuses on outlining our theses on four verticals that we’re particularly excited about in 2022: artificial intelligence; fintech; cybersecurity; and healthtech.
Artificial intelligence has remained a super-hot category for good reason: It has the potential to transform nearly every industry and business.
At Insight, we’ve long been bullish on the many use cases for AI. In the past year, we’ve invested in image recognition software from Netherlands-based ScreenPoint Medical, which improves early detections of breast cancer, Covera Health, which provides a quality analytics platform to reduce medical errors in radiology, CARTO, which helps companies use and understand spatial analytics, and Laminar, a cloud data security platform to continuously monitor and protect against data leaks – among other game-changing companies.
In total, Insight invested in 49 different companies across a broad spectrum of artificial intelligence and machine learning use cases in 2021, which represents a 172% increase from the year prior.
As we look ahead into 2022, we expect artificial intelligence tools to continue to dominate. We see the ecosystem dividing into three primary categories:
- Layer 1 - Base platform companies: Algorithms; frameworks; infrastructure; and workbenches for creating ML systems
- Layer 2 - Cross-industry capability companies: Turnkey machine learning-based systems that solve specific problems spanning multiple industries (e.g. cybersecurity)
- Layer 3 - Industry-specific companies: Applications powered by prediction or classification systems that target specific, niche use-cases in one domain
Companies and investors will find valuable AI/ML software across all three layers. At Insight, we initially focused on layers two and three. We invested in startups creating robust ML systems that addressed specific problems, either vertically (like credit underwriting company Zest AI) or horizontally (like cybersecurity company SentinelOne). We thought that economic moats were hardest to build at layer one, in part as a result of robust open-source ecosystems and because large public cloud vendors deliver many of these tools at low prices.
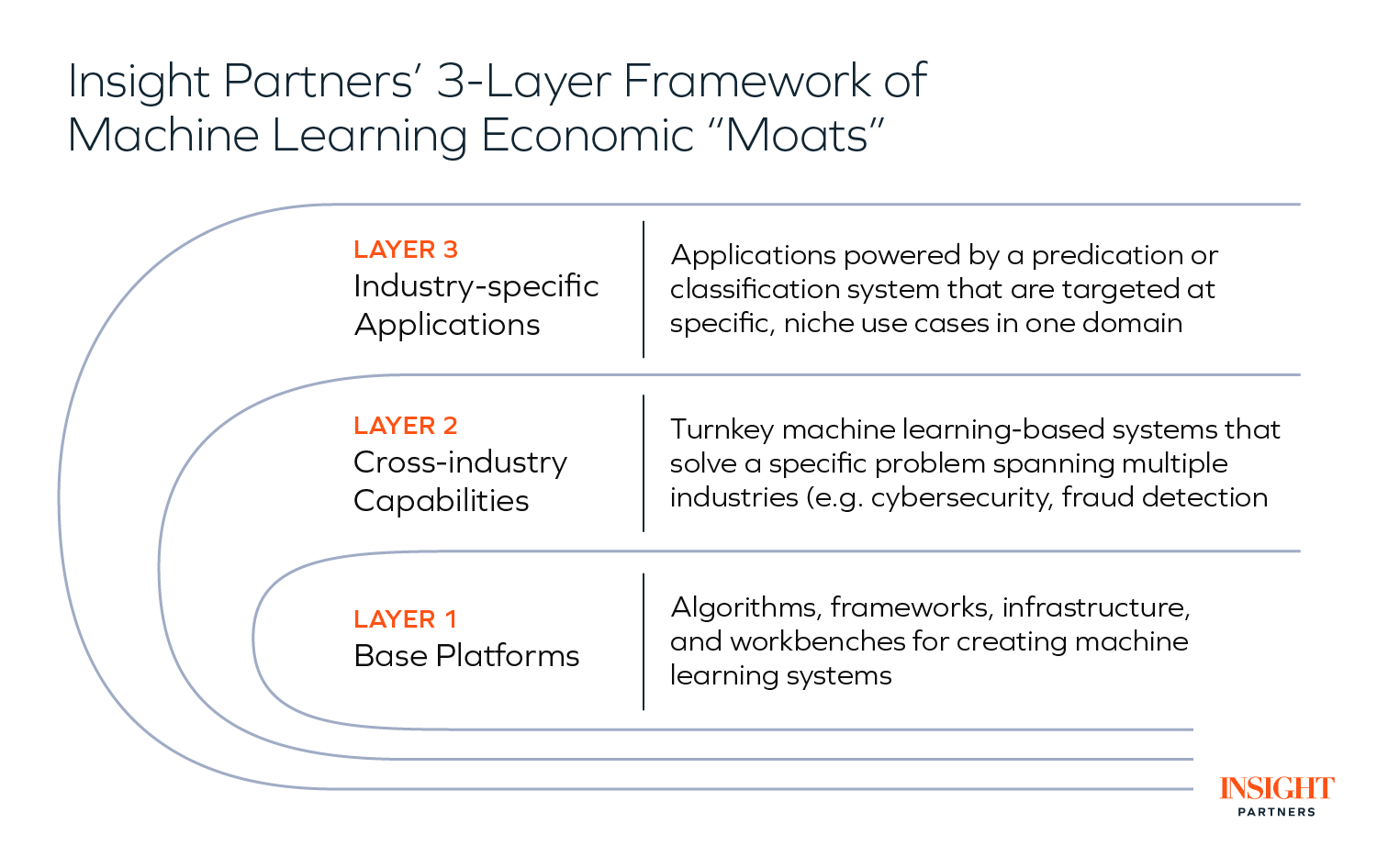
Insight Partners’ 3-Layer Framework of Machine Learning Companies
Throughout this year, however, we realized we were missing a piece of the puzzle. While there will always be market demand for cross-industry capabilities and industry-specific applications, 2021 taught us that as companies try to productize AI for the first time, base platforms will become increasingly important as well.
After all, more companies than ever are charging forward on artificial intelligence projects to try to guide business decisions and save time and money. A whopping 90% of enterprises are either actively running AI projects or plan to in the next 12 months, according to a recent survey.
But here’s the catch: Most of these projects will fail.
There are many reasons why, including a lack of sufficient data or black-box models that spit out indecipherable results. Regardless of the reason, high failure rates can hurt the ecosystem if they result in corporations’ hesitation to move forward with future AI projects.
Accordingly, Insight is particularly excited about a subsection of AI tools that can significantly increase companies’ likelihood of succeeding at their project goals: Machine Learning Operations or MLOps.
MLOps tools can improve a machine learning pipeline from start to finish by helping gather, manage, and label data, experiment and test the model selection, deploy multiple models in production at once, and protect against model and data drifts and attacks.
Holistically, the goal is to improve communication and collaboration between data scientists, data engineers, and business analysts through the machine learning lifecycle, similar to how DevOps tools help improve communication in the software development lifecycle. Machine learning is an iterative process with constant feedback loops and the need for continuous monitoring, which makes MLOps tools to manage this process even more critical.
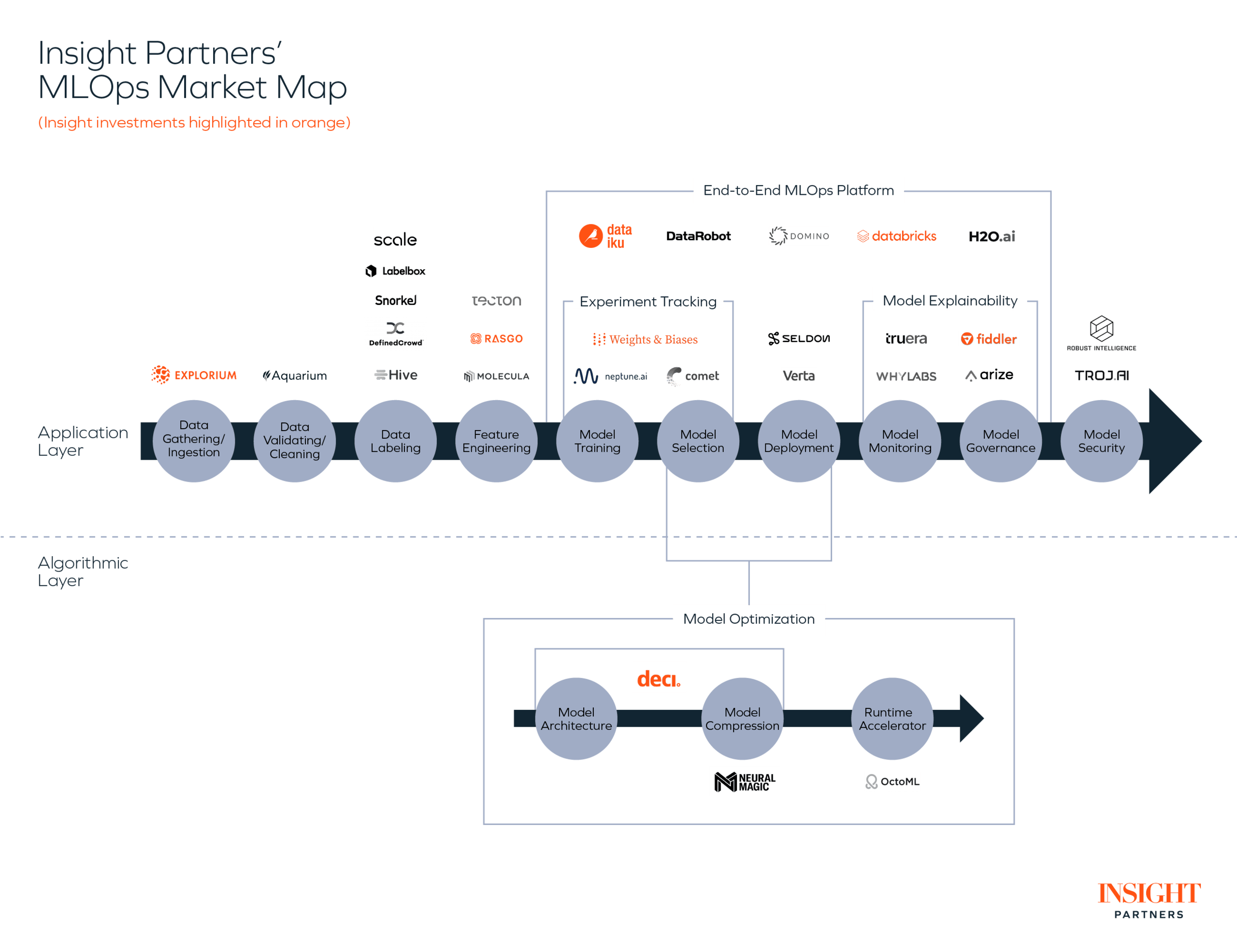
Overview of some of the key areas of an MLops pipeline
While every company rolling out AI projects can benefit from MLOps, the type of tools they require will depend on their needs, resources, and strategies.
Companies should ask themselves: How sophisticated is our data science team? How mission-critical do we want our models to be? Are our data sources structured or unstructured? Do we want open source, commercial, or homegrown tooling?
The answers to those questions will point companies to specific MLops tools that are best suited for their specific challenges.
For example, some platforms – like Dataiku or DataRobot – are end-to-end products with wide capabilities, which are typically easier to use and thus encourage model creation and experimentation from workers across job functions, regardless of technical knowledge. These so-called “citizen data scientists” can boost analytic workflows for companies with fewer data science experts and create significant value, but there's a risk that the deployed models are not as well understood or controlled. These types of tools are best for companies that want to use machine learning to drive business insights and analytics rather than as part of their core business function.
If a company has a sophisticated data science team, however, it may lean towards specific point solutions, or what Insight calls “best-in-class” MLOps solutions. While these products require greater technical knowledge to maintain and deploy, they allow for much more control, depth, and sophistication in a system. One startup offered the apt analogy that using platforms versus best-in-class solutions is like driving an automatic car versus a stick-shift.
There are multiple MLOps tools for each part of the pipeline, and we see a world where each tool has enough market space to support it becoming a large company.
At Insight, we spent 2021 learning about and investing in many of what we consider to be the best-in-class MLOps tools – as determined by highly satisfied customer feedback, clear market momentum, and well-respected and knowledgeable teams. This perspective led to our investments in Explorium, Rasgo, Weights & Biases, Deci, Run AI, Fiddler, Tonic, Dataiku, and Databricks, as well as several others that have yet to be announced.
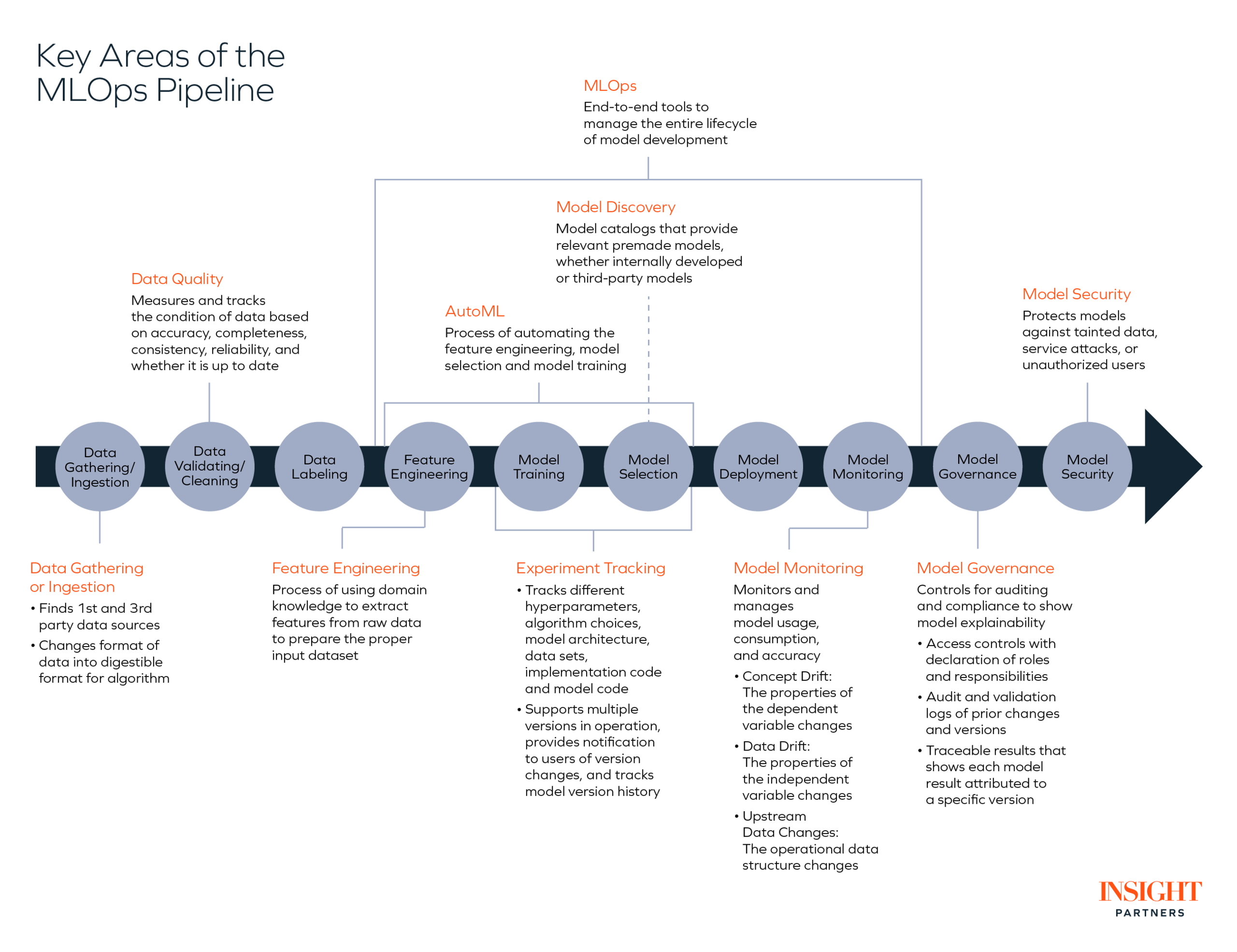
Insight Partners’ MLOps market map
While Insight expects MLOps to continue to be an essential driver of AI success in the new year, we also expect to see several other trends take shape in 2022:
- First, we will see the emergence of two machine learning pipelines, one for structured and one for unstructured data. Unstructured data sources – like images, videos, and audio – require specific data warehouses, data management, pipelines, and model management tools that maximize productivity and accuracy. Given the nuances and complexities between the two data types, we expect to see more MLOps tools shift towards owning their category for one or the other.
- As enterprises scale from dozens of models to hundreds or even thousands of models, we expect the increased industrialization of AI. This will result in the rise of an orchestration layers that sit on top of machine learning pipelines to help manage all the different tooling. These layers will integrate across multiple tools in the ML pipeline – whether open source, commercial, or homegrown – and provide an integrated environment (or so-called “single pane of glass”) to better track and manage ML pipelines. Orchestration layers will help enterprises gain more control over their pipelines while still supporting best-in-class tools.
- Insight believes we are at an inflection point of moving from a “model-centric” to a “data-centric” world. In a model-centric approach, you ask how you can change a model’s code to improve the performance of the system, whereas, in a data-centric model, you ask how you can change the data to improve it [system performance]. We expect the most successful tools of 2022 to be focused on supporting the new tasks, workflows, and jobs spawned by the data-centric movement.
- We will also see new class of MLOps tools focused on closing the growing AI skills shortage and gap across businesses. These tools will focus on increasing the efficiency of the model production process and lower the bar for ML development.
- Finally, we expect to see a significant increase in the overall enterprise adoption of ML, with a shift from experimentation to deploying models in full production. Last year, we saw many enterprises form AI research teams and hire data scientists to experiment with machine learning. In 2022, we believe these enterprises will start realizing the full capacity of their AI projects and driving significant business value. This increase in enterprise adoption will also be driven by the explosion of edge devices that will enable new use cases of ML adoption.
As active machine learning and artificial intelligence investors, we are excited to continue to watch the market and support new entrants in what will surely be another dynamic and exciting year.